Why AI Projects Fail — The Data Quality Crisis
Why your AI projects fail: the silent crisis of Data Quality
"Why isn't my AI project working?"
It's a question many CDOs, CIOs and Data Quality Managers are asking themselves today, after months of investment, testing and model training... for disappointing results.
And in over 70% of cases, it's not the algorithm that's to blame.
👉 It's the data.
According to several studies, 70% to 80% of AI projects fail- twice as many as traditional IT projects. And this rate continues to rise as AI is deployed in new business use cases.
In AI, one truth persists: garbage in, garbage out.
Data Quality: The Bedrock of AI
Artificial intelligence models simply reflect the data they are provided with. If this data is blurred, incomplete or biased, even the most advanced model will produce erroneous, unusable or dangerous results.
Here are the main flaws encountered in data-related AI projects:
- Inaccurate or incomplete data → inefficient learning
- Biased datasets → reproduction or amplification of discrimination
- Data scarcity or excess → under-learning or over-fitting
- Poorly labeled or compartmentalized data → inability to detect good patterns
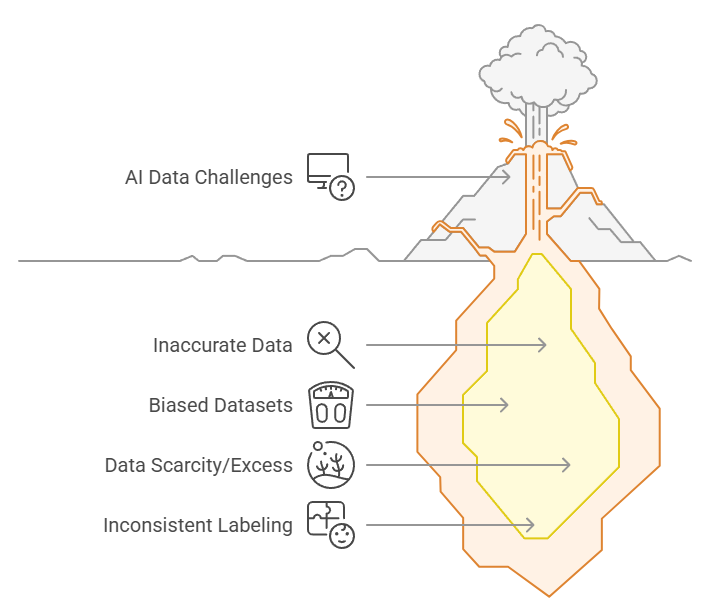
Case studies: when poor data quality causes AI to fail
🧪 IBM Watson for Oncology
Despite a $62 million investment from MD Anderson Cancer Center, the project failed to deliver useful oncology recommendations.
The cause: the model had been trained on hypothetical data, rather than actual patient records.
In addition, the opaque nature of the system's decisions - a "black box" - severely diminished physician confidence, leading to theproject's abandonment.
🧑💼 Amazon - AI recruitment tool
The recruitment tool developed by Amazon was trained on historical hiring data heavily biased in favor of men.
As a result, the algorithm systematically downgraded CVs mentioning female activities or groups, and valued formulations associated with masculine language.
After several attempts to correct the bias, the project was finally abandoned.
✈️ Air Canada - AI Chatbot
A customer received incorrect information on the refund policy via Air Canada's AI chatbot.
A court found the company legally responsible for the information provided by the bot, and forced it to honor the refund.
This case highlights the concrete legal risks associated with AI deployed on erroneous data.
📰 Apple Intelligence - News summary (2025)
In 2025, Apple deployed a generative AI system tasked with summarizing news articles.
The problem: the tool invented information and wrongly attributed it to credible sources such as the BBC.
In the face of controversy, Apple was forced to suspend the feature, and re-evaluate the way it tags AI-generated content.
The consequences of poor data quality
- Millions of euros wasted on development, training and infrastructure
- Months or years wasted in the wrong direction
- Loss of trust, both internally and with end-users
- Risks of non-compliance: RGPD, CSRD, e-invoicing, Basel III, etc.
How to guarantee the success of your AI projects: a solid data quality foundation
Organizations need to treat data as a strategic asset, and put in place a robust Data Quality framework even before building AI solutions.
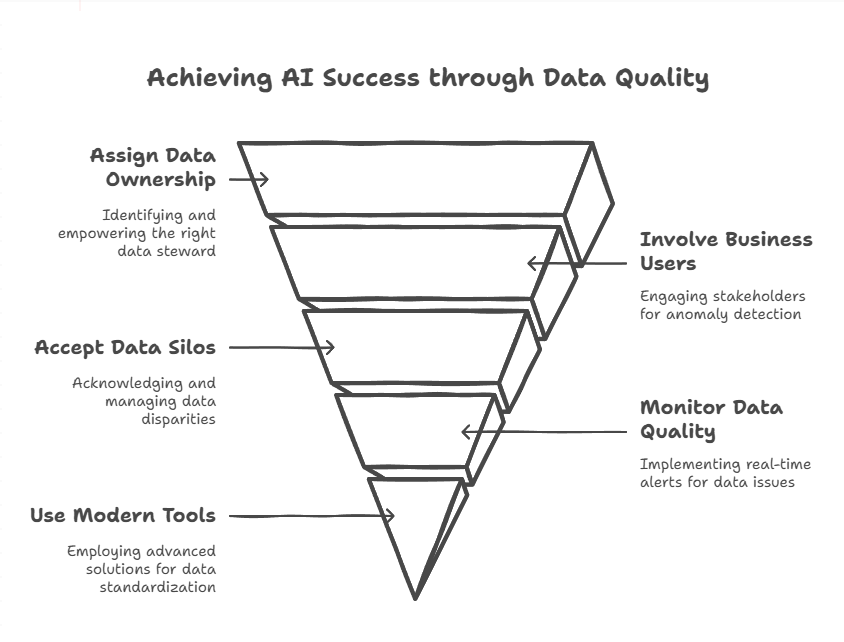
This involves:
- Assigning responsibility for data quality to the right person: this person is often already in the organization. This person must have in-depth business and data knowledge. These two pillars cannot be outsourced.
- Involve business users in data quality processes: they are the ones who best understand the anomalies that have a concrete impact on business operations.
- Accept that data silos are inevitable: they reflect the diversity of departmental uses and structures. Rather than trying to eliminate them, you need to use tools capable of reconciling data from heterogeneous sources in real time, upstream of AI algorithms.
- Monitor data quality continuously (Data Content Observability): we need an automatic monitoring capability that sends relevant, contextualized alerts to the right people as soon as a real risk appears.
- Use modern tools for standardization and remediation operations: abandon accumulated Python scripts, which are often opaque and difficult to maintain. The priority is readability, transparency and collaboration between business and data teams.
Are you launching an AI project? Start with the quality of your data.
At Tale of Data, we help companies secure their AI projects by building a robust foundation of data quality.
Our no-code platform enables you to :
- industrialize dataremediation,
- continuously monitor data quality (content observability),
- document each transformation,
- and empower business users without technical dependency.
With Tale of Data, your AI projects finally rest on a reliable, traceable and compliant foundation.
📅 Are you a CDO, CIO or leader of a strategic AI project?
Don't let your data compromise your results.
👉 Book an appointment for a personalized diagnosis
You May Also Like
These Related Stories
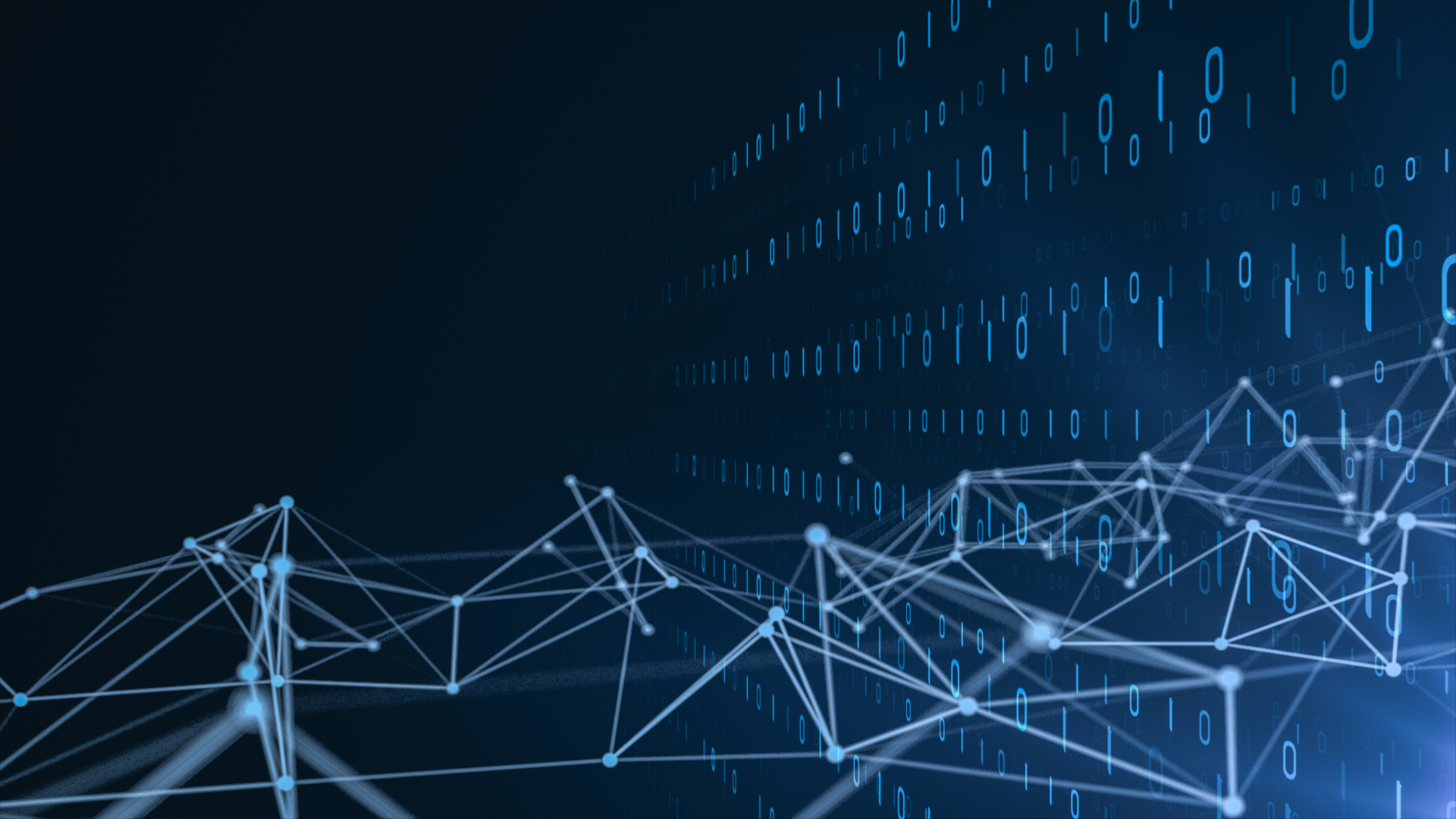
Generative AI and data quality: a virtuous circle for innovation
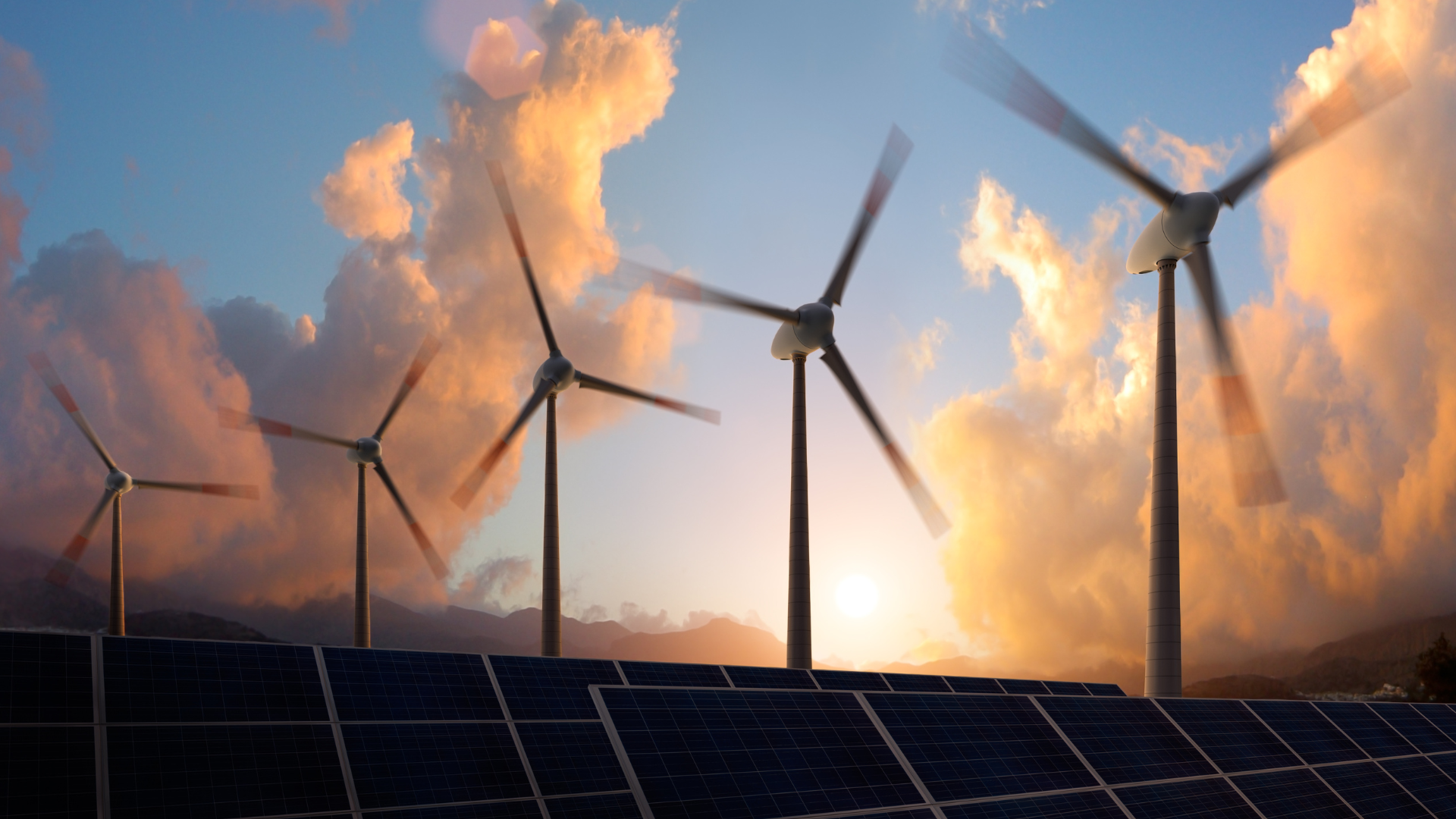
TotalEnergies customer testimonial
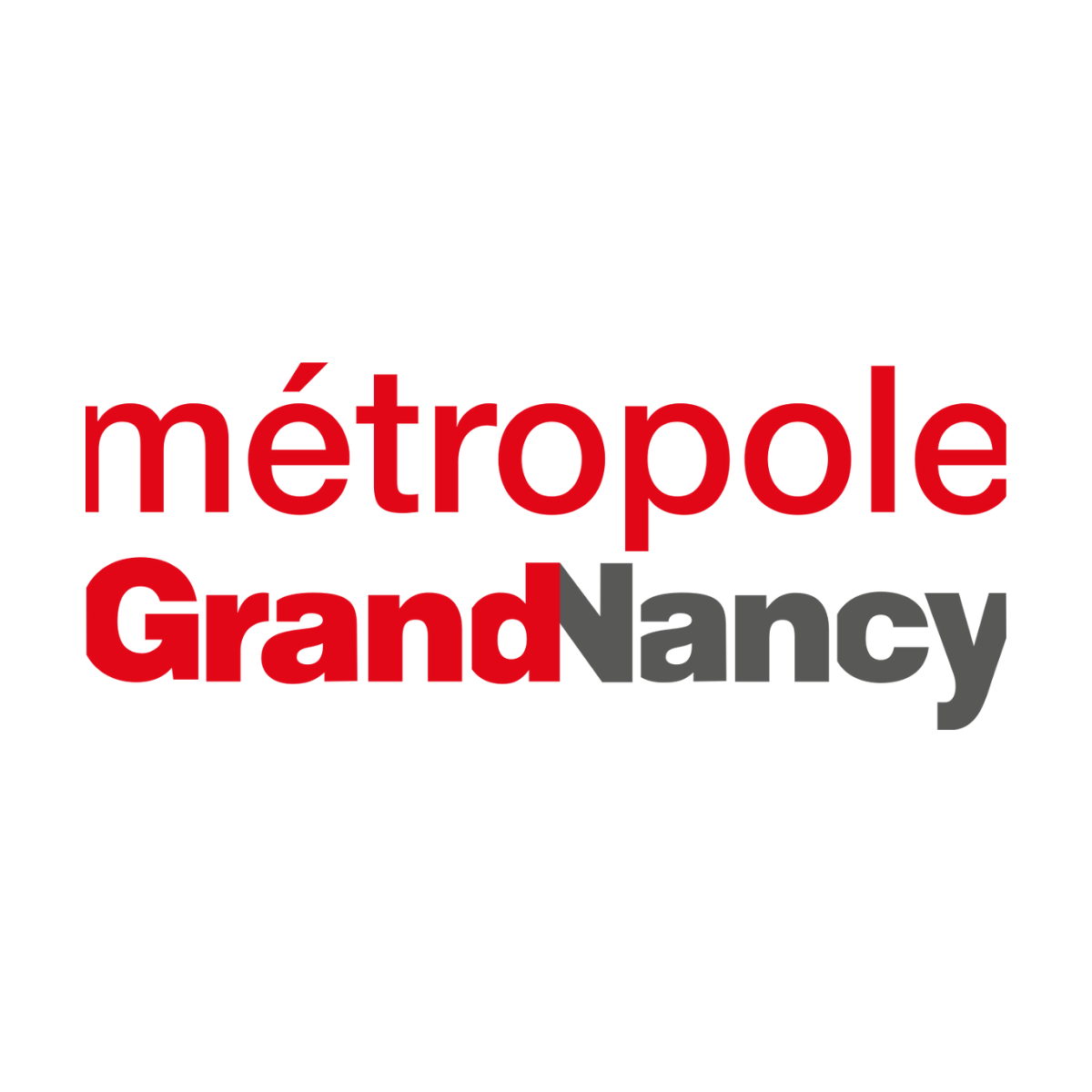